In some sense, it is with a heavy heart that I write my last permanent contributor blog post at the Duck. I’ve loved being with the Ducks these past years, and I’ve appreciated being able to write weird, often off the track from mainstream political science, blogs. ...
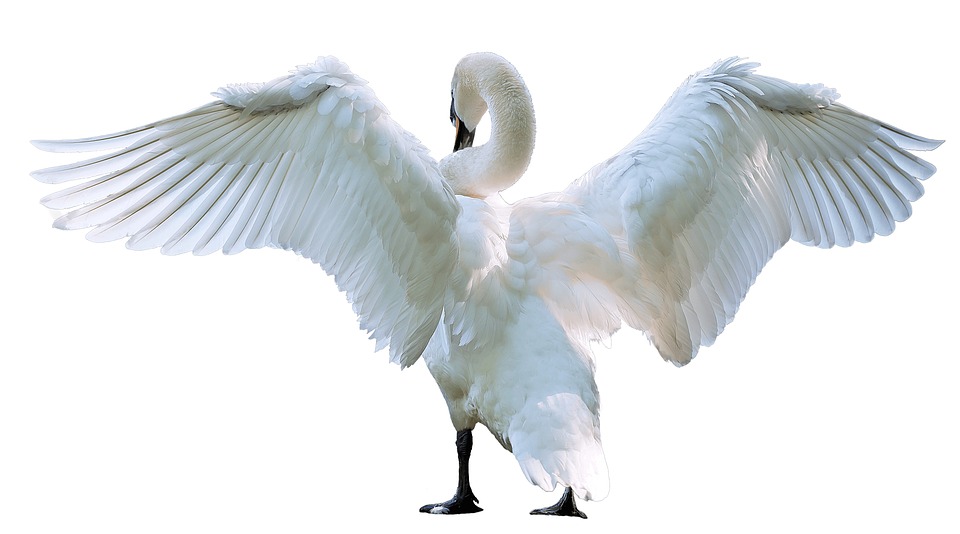